ELM: Embodied Understanding of Driving Scenarios
Hang Qiu3, Hongzi Zhu2, Minyi Guo2, Yu Qiao1, Hongyang Li1
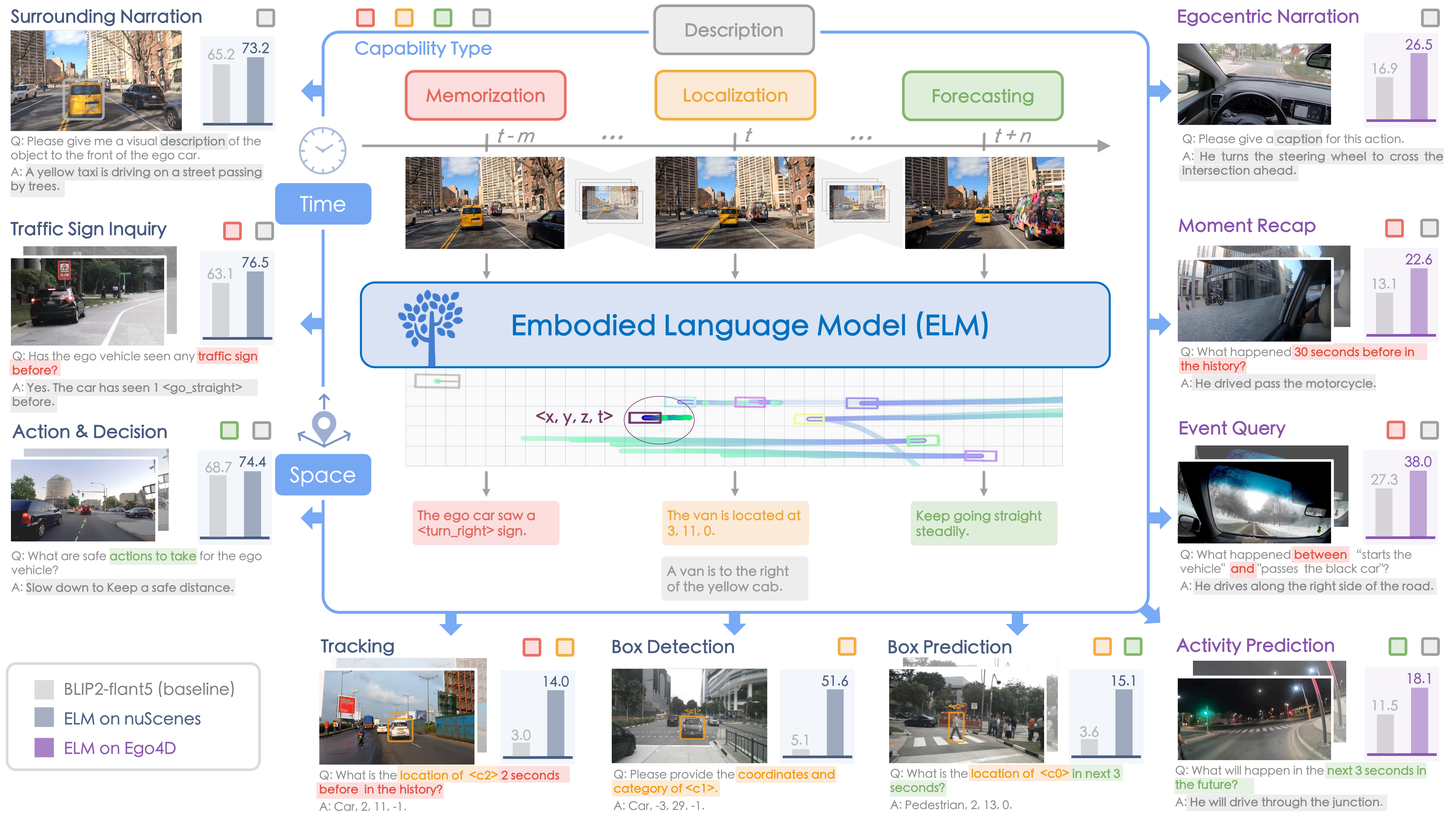
ELM is an embodied language model for understanding the long-horizon driving scenarios in space and time. Compared to the vanilla vision-language model (VLM) being confined to the scene description task, we expand a wide spectrum of new tasks to fully leverage the capability of large language models in an embodiment setting. ELM achieves significant improvements in various applications.
Abstract
Embodied scene understanding serves as the cornerstone for autonomous agents to perceive, interpret, and respond to open driving scenarios. Such understanding is typically founded upon Vision-Language Models (VLMs). Nevertheless, existing VLMs are restricted to the 2D domain, devoid of spatial awareness and long-horizon extrapolation proficiencies. We revisit the key aspects of autonomous driving and formulate appropriate rubrics. Hereby, we introduce the Embodied Language Model (ELM), a comprehensive framework tailored for agents' understanding of driving scenes with large spatial and temporal spans. ELM incorporates space-aware pre-training to endow the agent with robust spatial localization capabilities. Besides, the model employs time-aware token selection to accurately inquire about temporal cues. We instantiate ELM on the reformulated multi-faced benchmark, and it surpasses previous state-of-the-art approaches in all aspects.
Methodology
Model Overview
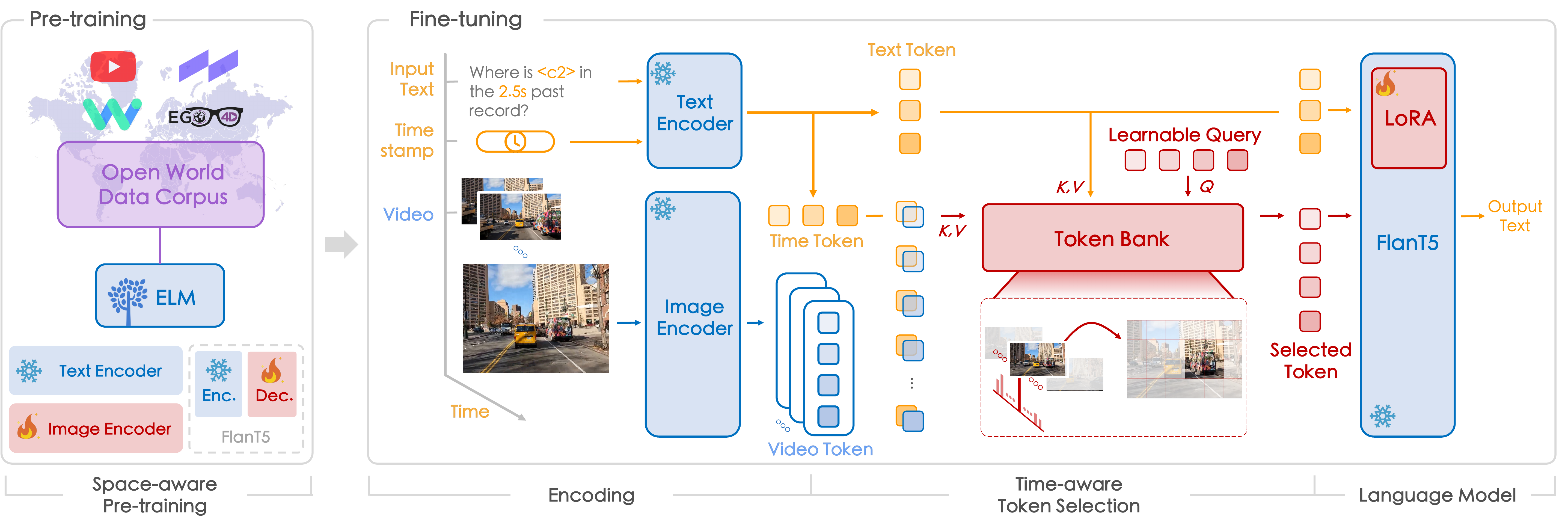
Systematic Pipeline of ELM. It consists of Pre-training by open-world data corpus and Fine-tuning on diverse tasks. To initialize the Space-aware Pre-training, we collect extensive image-text pairs from the world, empowering ELM with spatial localization while preserving the description ability in driving scenarios. In the fine-tuning process, the inputs to ELM are videos, timestamps, and text prompts. After encoding the inputs into tokens, ELM leverages the proposed Time-aware Token Selection to gather the appropriate tokens as instructed by prompts. Finally, the tokens are sent to the language model to generate output texts.
Space-aware Pre-training
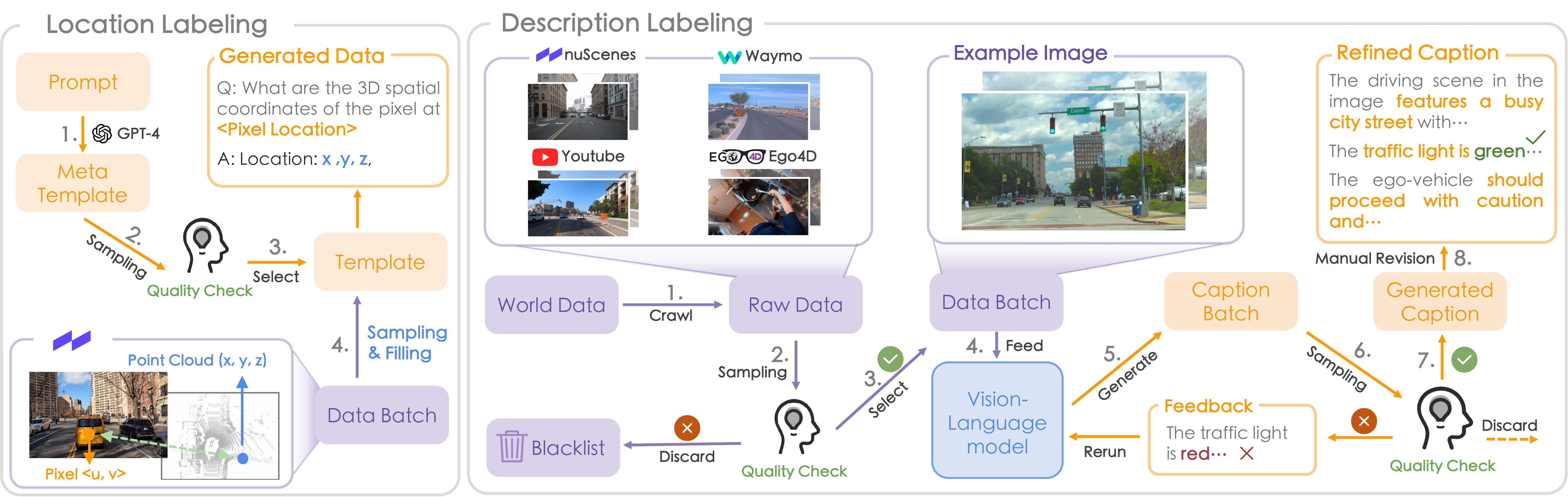
Annotation workflow with human quality check in the loop. For location labeling: we first select diverse templates from the GPT generated candidates. Pixel-point pairs as annotated in the nuScenes are then sampled and filled into the templates to form our location pre-training data.For description labeling: Node 4 utilizes LLaMA-Adapter V2 to obtain diverse labels on nuScenes, Waymo, YouTube, and Ego4D with predefined prompts. Two rounds of quality check are conducted in Node 3 and 7 by inspectors to guarantee the image and caption quality.
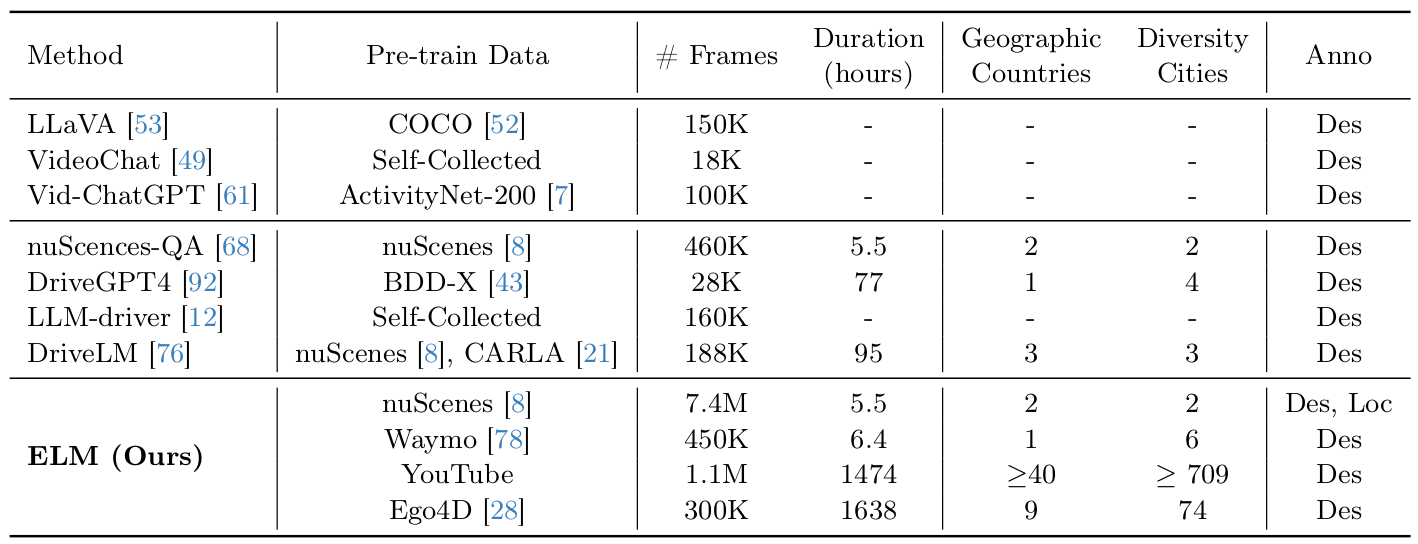
Statistics of pre-training data and comparison of data collection with other VLMs. Our pre-train data surpasses that in general vision (top) and autonomous driving (middle) in terms of quantity and diversity. Anno: the type of annotations; Des: description; Loc: localization.
Experiments
Visualization on the benchmark. We provide results for ten tasks and E2E planning through videos and corresponding QA pairs.
Results
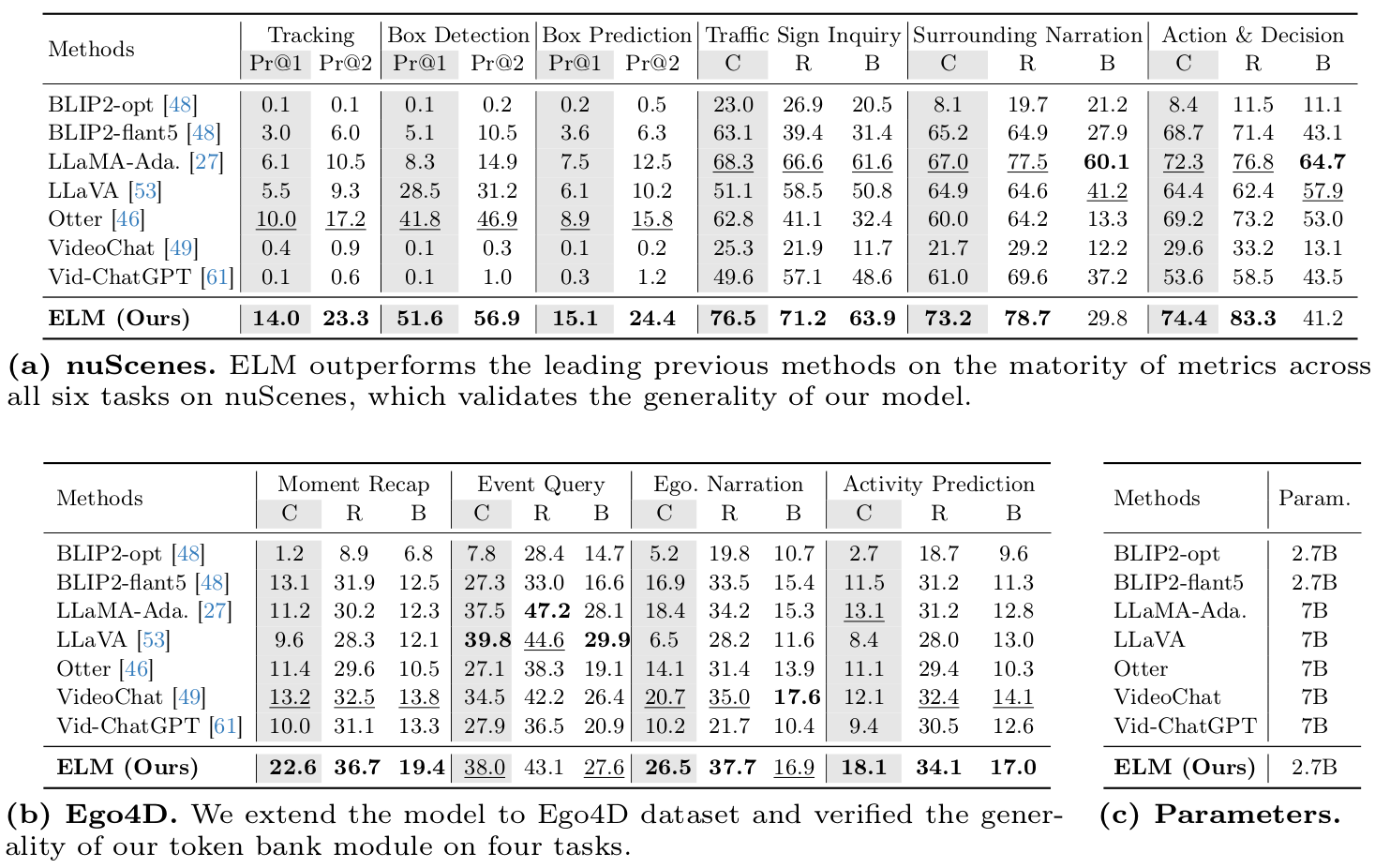
Comparison to State-of-the-arts. All methods are fine-tuned on the corresponding tasks. The main metrics (%) are marked in gray. Bold emphasizes top method; underline marks the runner-up. C: CIDEr; R: ROUGE-L; B: BLEU.
Citation
@article{zhou2024embodied,
title={Embodied Understanding of Driving Scenarios},
author={Zhou, Yunsong and Huang, Linyan and Bu, Qingwen and Zeng, Jia and Li, Tianyu and Qiu, Hang and Zhu, Hongzi and Guo, Minyi and Qiao, Yu and Li, Hongyang},
journal={arXiv preprint arXiv:2403.04593},
year={2024}
}